Intelligent microfluidics: From automation to decision-making
Introduction to intelligent microfluidics
Microfluidic devices, known for their exceptional precision in manipulating minute fluid volumes, have undergone a transformative evolution in recent years. Initially designed as passive systems, these devices have now emerged as dynamic, intelligent microfluidics through the integration of artificial intelligence (AI). By combining the microfluidic capabilities of precise fluid manipulation with AI’s advanced analytical and adaptive power, these systems can perform various functions. This fusion of technologies enables advanced functionalities, allowing microfluidic platforms to analyze complex biological and chemical processes in real-time, optimize workflows, and dynamically respond to changing conditions [1] (Figure 1).
This review explores the integration of AI into microfluidic systems, enhancing automation, real-time analysis, and decision-making. It highlights applications in diagnostics, drug testing, and cell sorting while addressing challenges like AI transparency and data integration.
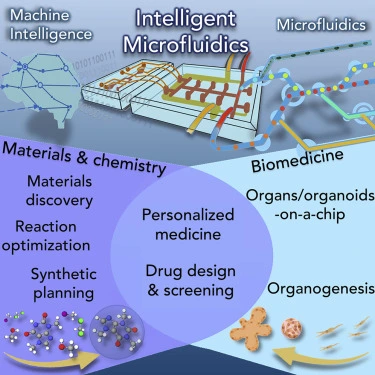
History of intelligent microfluidics
Microfluidics has played a transformative role in biomedical research by enabling precise fluid manipulation at the microscale. The concept of the laboratory-on-a-chip (LOC), introduced in the late 20th century, laid the foundation for miniaturized analytical systems capable of performing complex biochemical assays with minimal reagent consumption. Key advancements, including the development of micro total analysis systems (µTAS) and the incorporation of 3D printing for rapid prototyping, have expanded the flexibility and efficiency of these systems [3].
Simultaneously, machine learning (ML) has evolved into a powerful tool for data analysis, automation, and decision-making across scientific disciplines. Integrating these two fields has led to the rise of “intelligent microfluidics,” where AI-driven systems autonomously analyze and optimize experiments, enhancing efficiency and expanding the possibilities for biomedical research (Figure 2) [4].
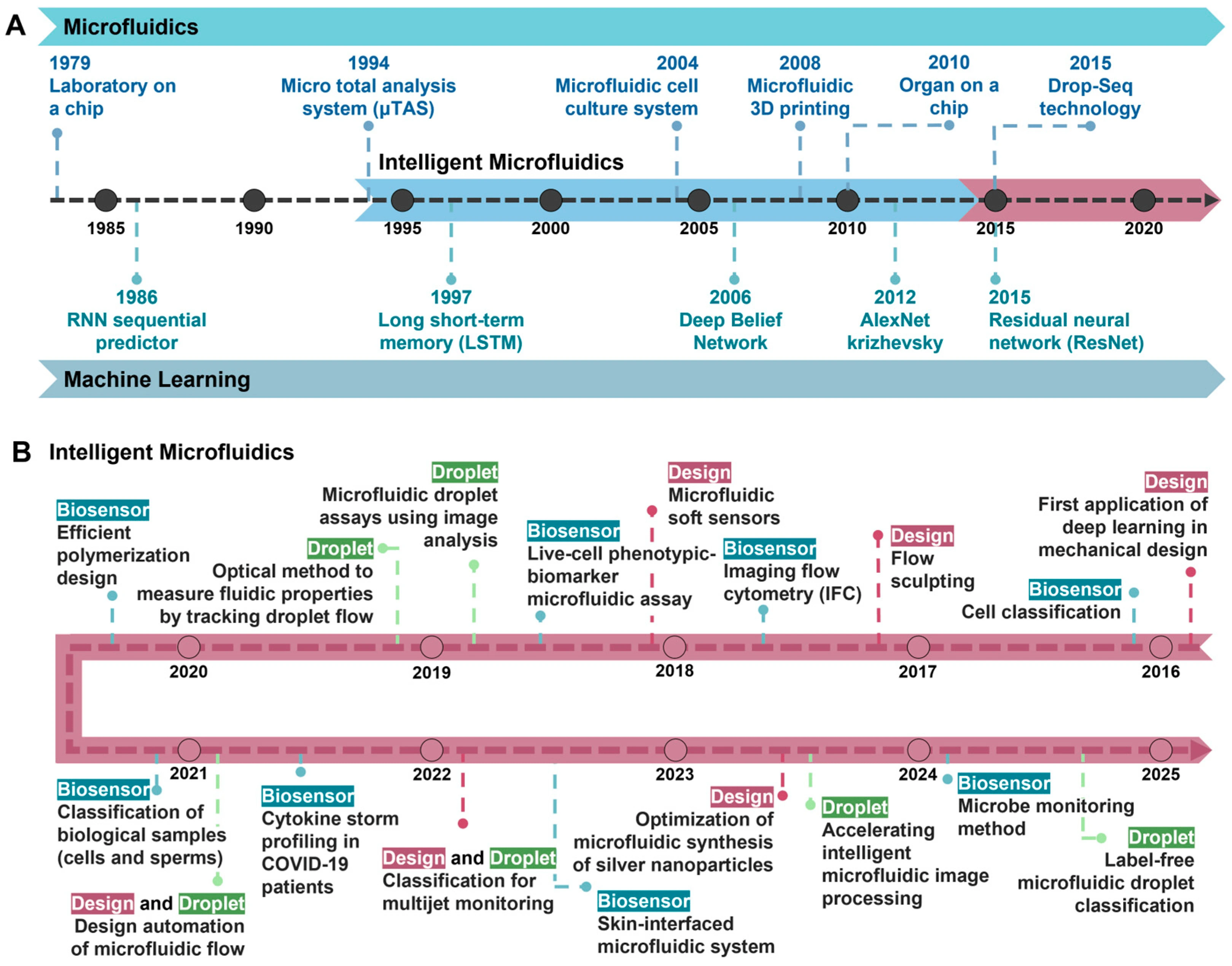
Fundamentals of AI
Foundational AI techniques, along with machine learning (ML) and deep learning (DL), form the core of modern AI, offering powerful methods for solving complex problems by enabling systems to learn from data and improve their performance over time (Figure 4).
Foundational AI techniques
Effectively selecting and applying foundational AI models is crucial for solving five key problem types. The regression problem is based on predicting continuous value outcomes, such as estimating cancer cell invasion probability based on tumor size and genetic markers. Classification assigns data into predefined groups using labeled data, aiding tasks like cell sorting [5], whereas clustering discovers hidden structures or clusters within data without predefined labels, which is useful for identifying gene expression patterns in genomics.
Dimensionality reduction helps simplify high-dimensional data while retaining key information, using methods like principal component analysis (PCA) or t-distributed stochastic neighbor t-SNE [6]. Finally, reinforcement learning focuses on training an agent through trial and error to maximize rewards in decision-making environments [7].
Machine learning and deep learning
Machine learning (ML) is a class of AI-based methods that direct computers to learn rules from data and then use the experience to improve their performance without explicit programming [8]. Deep learning (DL) realizes the feature extraction of input data from low- to high-level by establishing and simulating the human brain’s neural structure for information processing. This allows the machine to understand and learn from the data and then obtain information [9] (Figure 3).
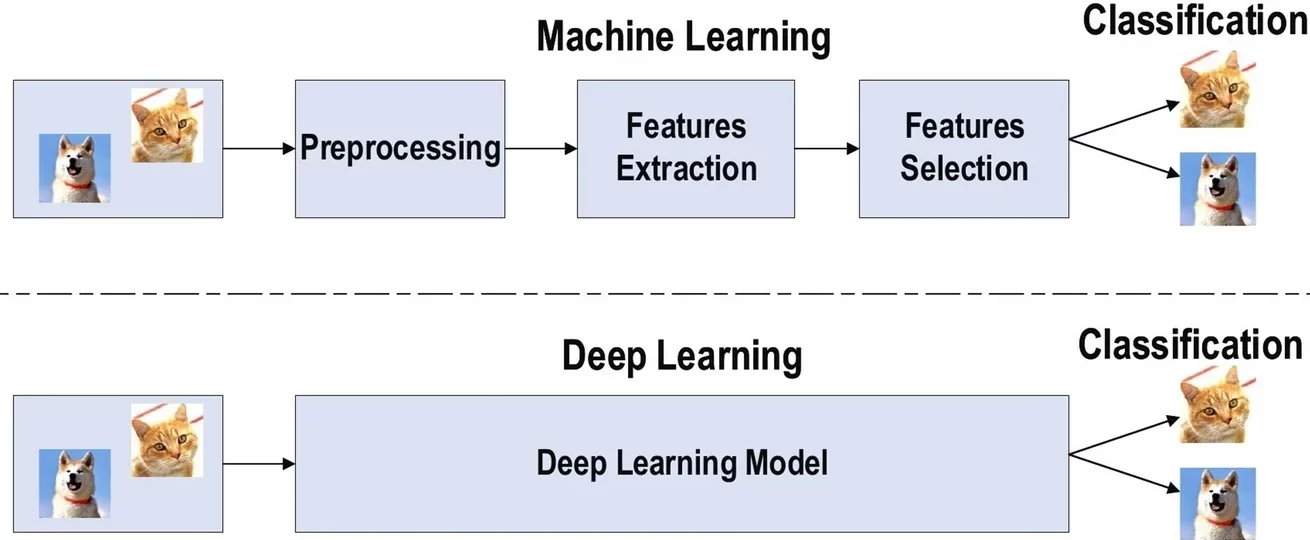
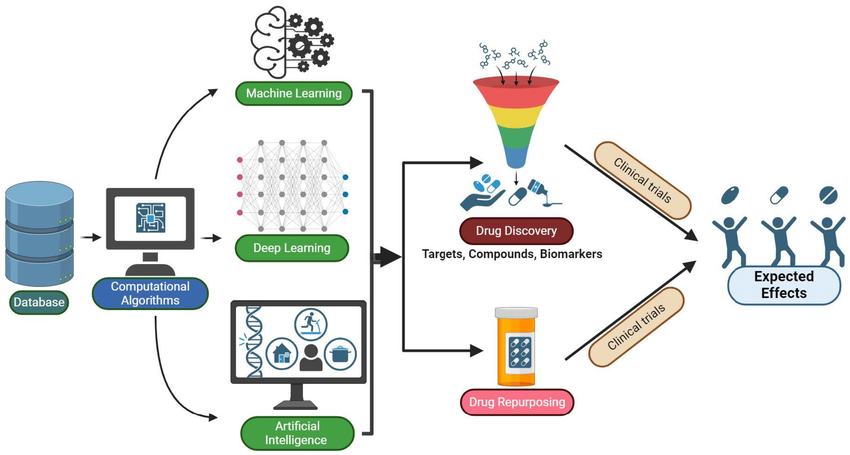
Intelligent microfluidics benefits
The integration of AI into microfluidic systems offers a range of transformative benefits that significantly enhance both functionality and efficiency (Figure 5).
- AI-driven simulation: AI learns patterns from data and establishes the association between features and data. It can be pre-trained to predict fluid dynamics faster than traditional computational fluid dynamics (CFD) models [12]. It can solve problems that are hard or next to impossible for traditional methods.
- Generative design: AI can optimize and generate innovative microfluidic chip designs that maximize performance while minimizing material use [12].
- Scalability and reproducibility: AI ensures standardized and high-throughput microfluidic processes.
- Real-time monitoring: Intelligent microfluidics can analyze sensor data to adjust flow rates, pressures, or temperatures dynamically [12].
- Process automation: Microfluidic systems and the introduced AI models can provide feedback to each other, which leads to model optimization and the automation and intelligence of microfluidic systems [13].
- Increased efficiency and precision: Faster, more accurate experiments with minimal human intervention and reduced need for extensive expertise.
- Pattern recognition: AI can identify trends in complex biological or chemical data collected from microfluidic devices.
- Predictive analytics: DL utilizes large amounts of data for feature extraction, such as the classification and prediction of data from microfluidic systems [14]. It can forecast experimental outcomes, optimize protocols, and reduce trial-and-error [12].
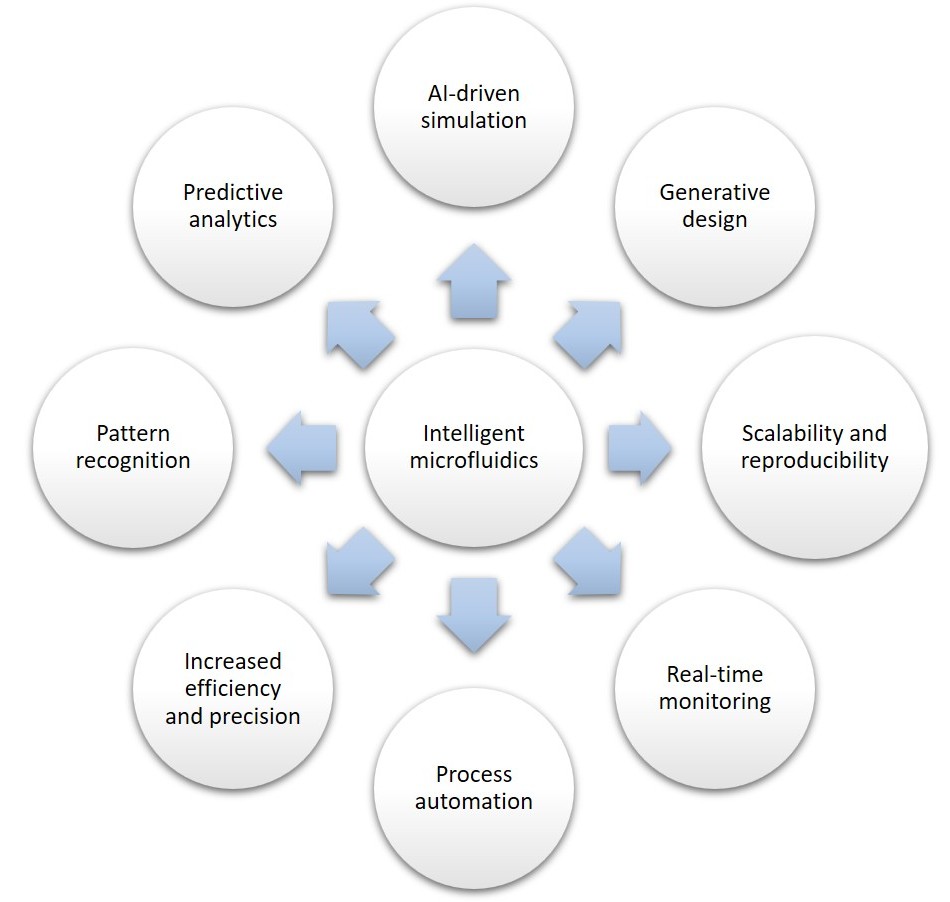
Key applications of intelligent microfluidics
AI microfluidics improve efficiency, precision, and scalability in different applications, such as flow control, droplet manipulation, point-of-care diagnostics, drug susceptibility testing, and cell sorting.
Flow control
Micropumps, which often consist of microvalves or piezoelectric valves, are crucial components in microfluidic devices. Their ability to seamlessly integrate into microdevices makes them essential for developing micro-total analysis systems (μTAS), which aim to perform complex laboratory processes on a miniaturized chip (Figure 6).
Abe et al. applied reinforcement learning (RL) to optimize the timing of valve operation in peristaltic micropumps, improving their maximum flow rate. This demonstrates how intelligent microfluidics can enhance fluid transport efficiency [15]. Shayan et al. designed a fully programmable biochip by integrating multiple microvalves, allowing for dynamic fluid movement control. This model enables real-time status monitoring and detection of potential security threats, such as unauthorized modifications or attacks on biochemical assays [16].
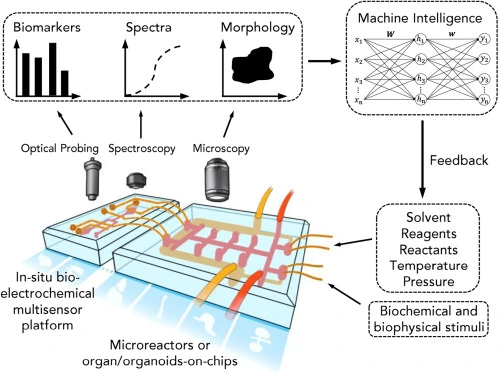
Droplet manipulation
Another important application of intelligent microfluidics is droplet manipulation. Precise control and minimization of flow rate variations are crucial for producing microdroplets of desired sizes. Mahdi and Daoud showed that an artificial neural network (ANN) could effectively predict microdroplet size [17], while Lashkaripour et al. developed an ML-based tool for designing single- and double-emulsion droplets, predicting key parameters like size and stability [18]. The tool can also automate the microfluidic chip design for a specific droplet size desired by the user (Figure 7) [19].
Zhang et al. were able to design inlets for customized concentration gradients, improving control over concentration distribution [20]. Beyond classification and design, incorporating ML techniques enables dynamic adjustments in microfluidic platforms by integrating real-time data for precise droplet manipulation. For instance, flow rate and concentration were predicted from microdroplet images by a trained deep NN [21].
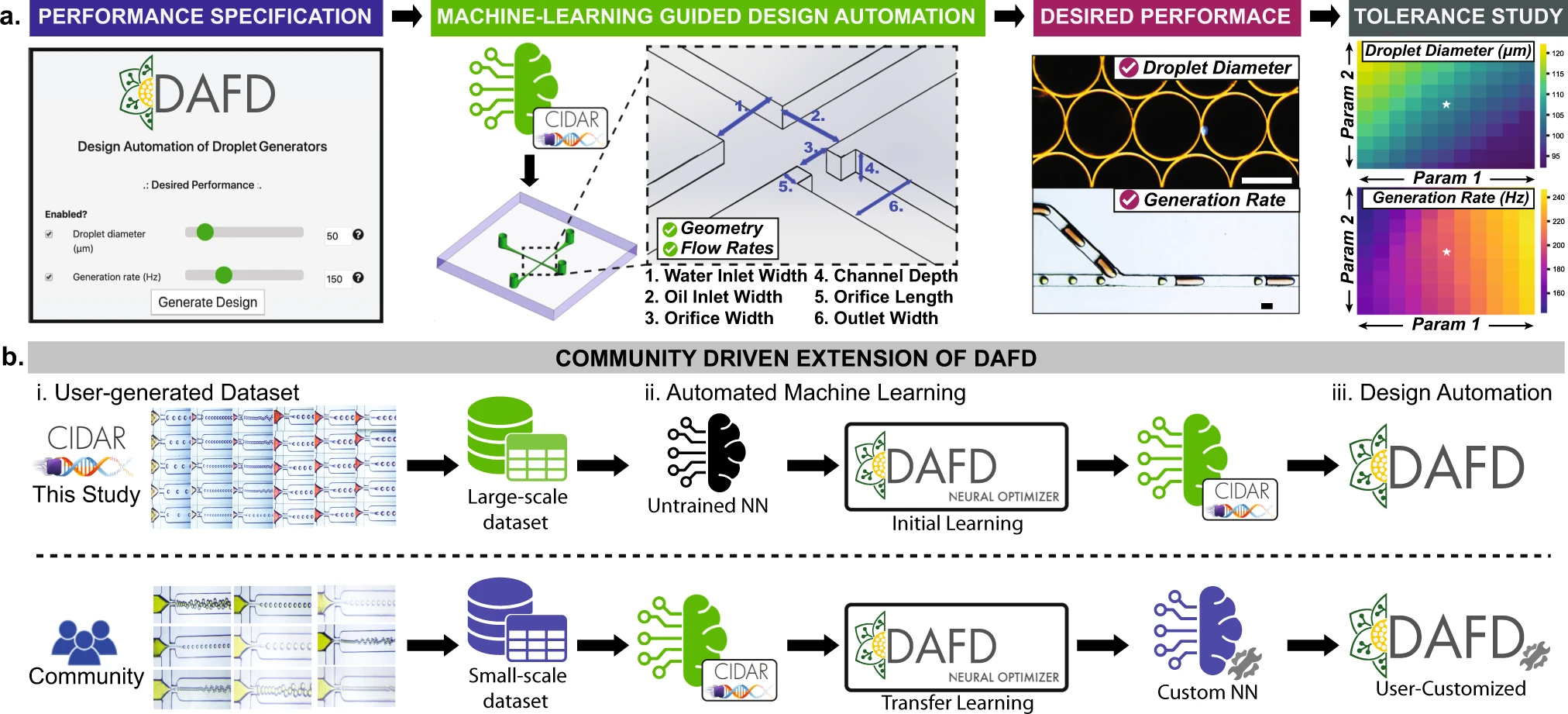
Point-of-care diagnostics and personalized medicine
AI microfluidics is transforming point-of-care testing (POTC) and personalized medicine by enabling faster, more precise, and automated biomedical solutions [22]. A compelling example is the work by Ao et al., who developed an automated intelligent microfluidics platform for tracking T cell dynamics and cytotoxicity within 3D tumor cultures. This system assessed treatment effectiveness and identified an epigenetic drug that enhanced T-cell infiltration into tumors, ultimately improving treatment efficacy in vivo [23].
ML further strengthens biomedical sensing applications, particularly in image classification and recognition. Munoz et al. developed an ML-based approach to detect label-free DNA using fractal structures in subnanoliter droplets following loop-mediated isothermal amplification (LAMP) [24]. Similarly, a semi-supervised tool has been used to enhance single-nucleus RNA sequencing by filtering out optical debris [25].
Additionally, optical immunoassay readouts have been optimized through AI, such as Song et al.’s digital enzyme-linked immunosorbent assay (ELISA) microarray, which enables rapid multiplex cytokine quantification in cancer patients undergoing therapy, reducing assay turnaround time and improving diagnostic efficiency [26]. Moreover, wearable soft sensors enable the measurement of pressure, force, and strain. ML algorithms assist in sensor calibration while also enabling the tracking and prediction of human movements and gait patterns (Figure 8) [27].
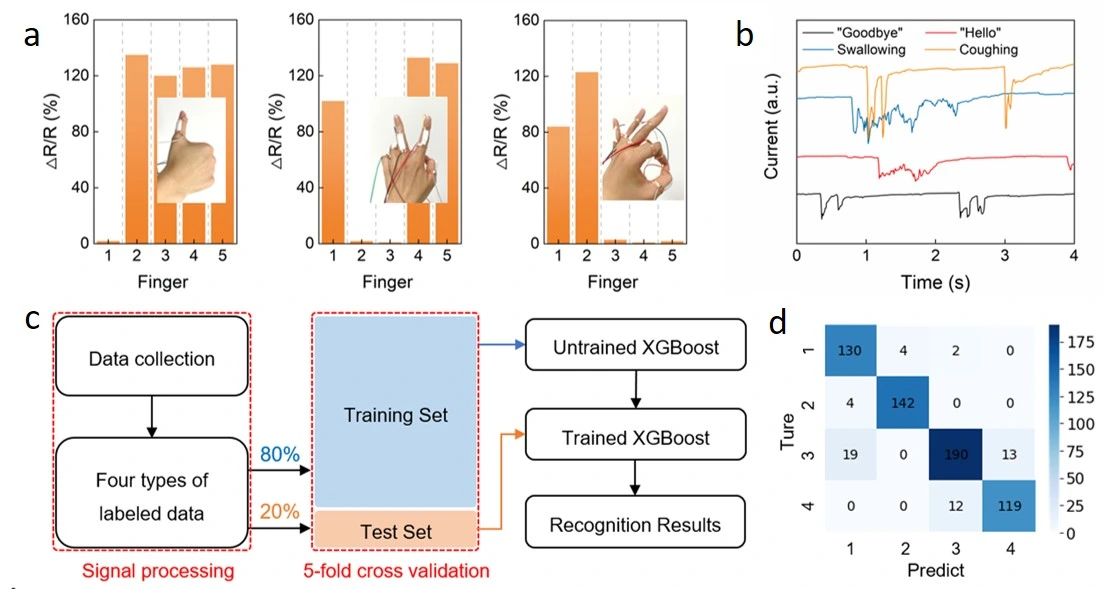
Drug susceptibility testing (DST)
Intelligent microfluidics plays a crucial role in cell analysis by not only isolating target cells but also assessing their susceptibility to pharmaceutical agents. Zhang et al. integrated convolutional neural networks (CNNs) for DST by predicting tumor viability based on morphological and optical changes [28]. Zhou et al. developed an intelligent platelet aggregate (activated by different agonist types) classifier that combines microfluidic flow cytometry, time-stretch microscopy, and CNNs (Figure 9). The tool can help in the choice of drugs for antiplatelet therapy [29].
Additionally, AI microfluidics combined with optical detection allows for rapid bacterial detection, screening of effective antibiotics, and determination of therapeutic ranges [30]. Moreover, DL addresses the issue of conventional imaging with 3D tissue models, by analyzing complex patterns in 3D images. These approaches enable precise, automated drug evaluation and may reduce the need for animal testing.
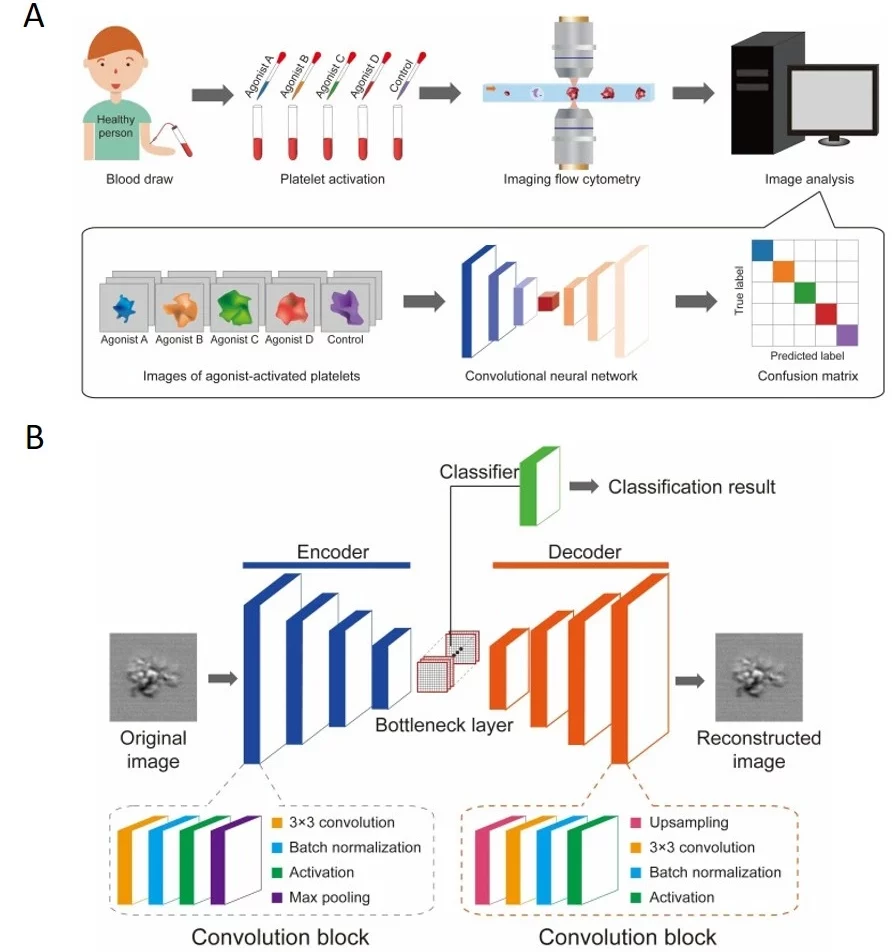
Cell counting and sorting
Intelligent microfluidics enhances cell counting, classification, and tracking by integrating optical, electrical, and impedance-based detection methods. ML algorithms can extract cell features from various imaging techniques (Figure 10).
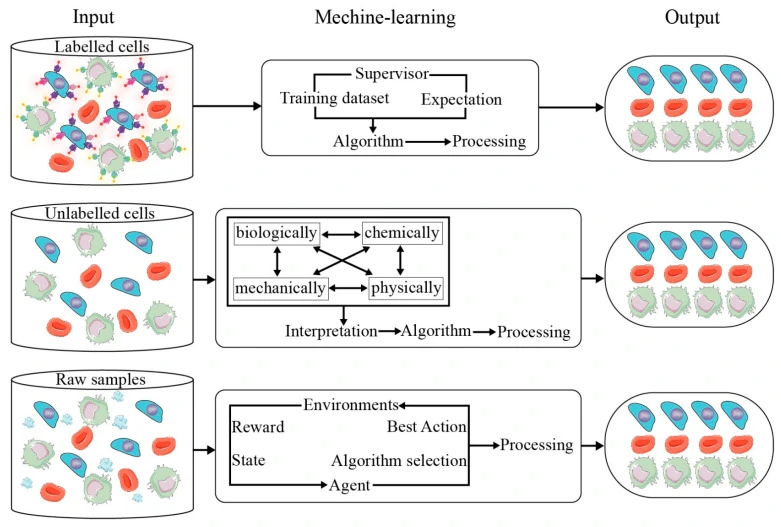
As an example of AI microfluidics, Wu et al. combined high-speed time-stretched microscopy with CNNs to classify thousands of cells (Leukemia cells, RBCs, algae, and platelets) per second with over 96% accuracy (Figure 11) [32]. Uslu et al. used magnetic immunoseparation to identify leukemia cells with a 91.6% precision [33]. Additionally, ML can distinguish subtle optical differences in cells, such as in CD4 and CD8 T lymphocytes or non-small-cell lung cancer using polarization microscopy [34].
With the use of microfluidic impedance flow cytometry, Feng et al. classified five distinct cancer cell types based on membrane capacitance, cytoplasm conductivity, and radius, with an accuracy of 91.5% [35]. Heo et al. introduced the real-time moving object detector (R-MOD) system for label-free imaging flow cytometry that enables real-time analysis as cells flow through a microfluidic channel. The system demonstrated a high processing speed of 500 fps and a mean average precision of 93.3% [36].
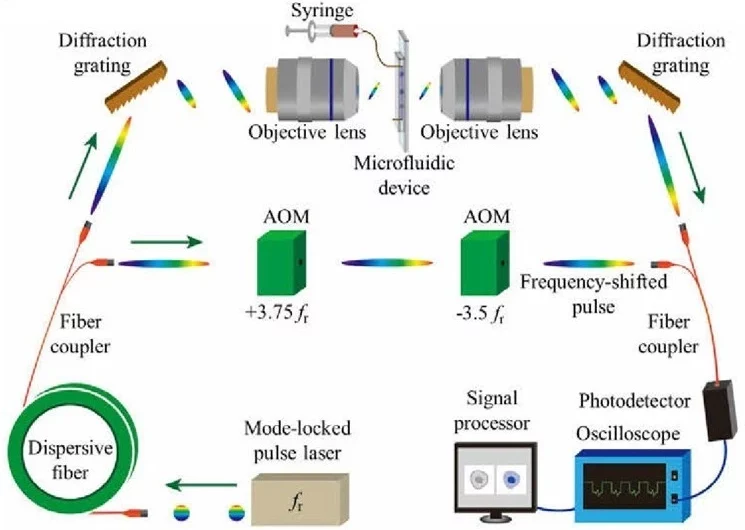
MIC projects using intelligent microfluidics
ElectroMed
The ElectroMed (Electrochemically-enabled high-throughput peptidomics for next-generation precision medicine) project aimed to develop a high-throughput peptide microarray technology for the screening of peptide sequences in cancer vaccines (GA No 862539). The project integrated electrochemical peptide synthesis and FinFET (Field Effect Transistors) sensors for real-time in situ protein detection. The tool was optimized through AI-based analysis to process complex biological data. AI contributed to enhanced data processing and high-performance acquisition, contributing to the creation of a cost-effective, programmable in situ screening tool for precision medicine.
NAP4DIVE
The current NAP4DIVE (Non-Animal Platform for Nanoparticle-Based Delivery across the blood-brain barrier Interface with Vehicle Evolution) project aims to develop an in vitro blood-brain barrier (BBB) model to test nanoparticle designs for drug delivery across the human BBB. The BBB-on-chip model will be validated before testing the drug delivery in established animal models (GA No 101155875). The project will integrate machine learning to predict the best nanoparticle candidates, reducing costs and animal testing. The initiative also seeks to enhance drug development efficiency and offer new treatment options for brain diseases like Parkinson’s and Alzheimer’s.
LOCAI
The LOCAI (Cell growth in lab-on-a-chip devices controlled by Artificial Intelligence) project aimed to develop a self-regulated microfluidic cell culture system that controls the growth parameters in real-time using AI based on image analysis. The concept behind the project was to let AI lead the model design. At the end, this concept was not achieved.
Challenges and future directions
The integration of ML into microfluidic systems is set to revolutionize fields like healthcare and synthetic biology. Advanced algorithms, including reinforcement learning and generative models, will improve system adaptability and autonomy by enabling real-time adjustments based on previous data. In medicine, AI microfluidics hold great potential for personalized treatment by continuously monitoring biomarkers, allowing precise and timely therapeutic interventions that enhance patient outcomes. The role of AI in droplet-based microfluidics is expected to expand, with future research focusing on reinforcement learning and generative models to optimize real-time decision-making and droplet manipulation and expand the scope of microfluidic applications [37].
Several challenges must be addressed when integrating AI into microfluidic systems. One key issue is the lack of transparency in AI models, often perceived as “black boxes.” To ensure reliability, particularly in medical applications, it is essential to enhance explainability and validation while maintaining strict quality control and risk management. Another challenge involves data volume and integration, as AI performance depends on the quality and scale of training datasets. Merging microfluidic automation with data from existing methods can be complex, but data fusion techniques may offer a more unified and accurate predictive framework. Encouraging collaborative data sharing and federated learning within the scientific community could further enhance the effectiveness and accessibility of AI-powered microfluidic systems [38].
Conclusion - Towards decision-making intelligent microfluidics
Intelligent microfluidics is transforming a wide range of applications, from flow control and droplet manipulation to diagnostics and drug susceptibility testing. By integrating advanced machine learning techniques, microfluidic systems are becoming more autonomous, precise, and adaptable, enabling real-time data analysis and optimization and offering faster and more efficient diagnostics and therapeutic monitoring solutions. Despite these promising developments, several challenges must be addressed. Key concerns include improving the transparency and interpretability of AI models, ensuring high-quality data integration, and optimizing computational efficiency. As AI continues to evolve, its synergy with microfluidics will drive further innovations, aiming for more advanced, scalable, and accessible lab-on-a-chip technologies across various scientific and medical fields.
Funding and Support
This review was written under the European Union’s Horizon research and innovation program under HORIZON-HLTH-2024-TOOL-05-two-stage, grant agreement No 101155875 (NAP4DIVE),
the European Union’s Horizon 2020 FETOPEN under grant agreement No 862539 (ElectroMed),
and the French National Research Agency (ANR) & the German Federal Ministry of Education and Research (BMBF) in the frame of Locai project.
This review was written by Celeste Chidiac, PhD.
Published in March 2025.
Contact: Partnership@microfluidic.fr
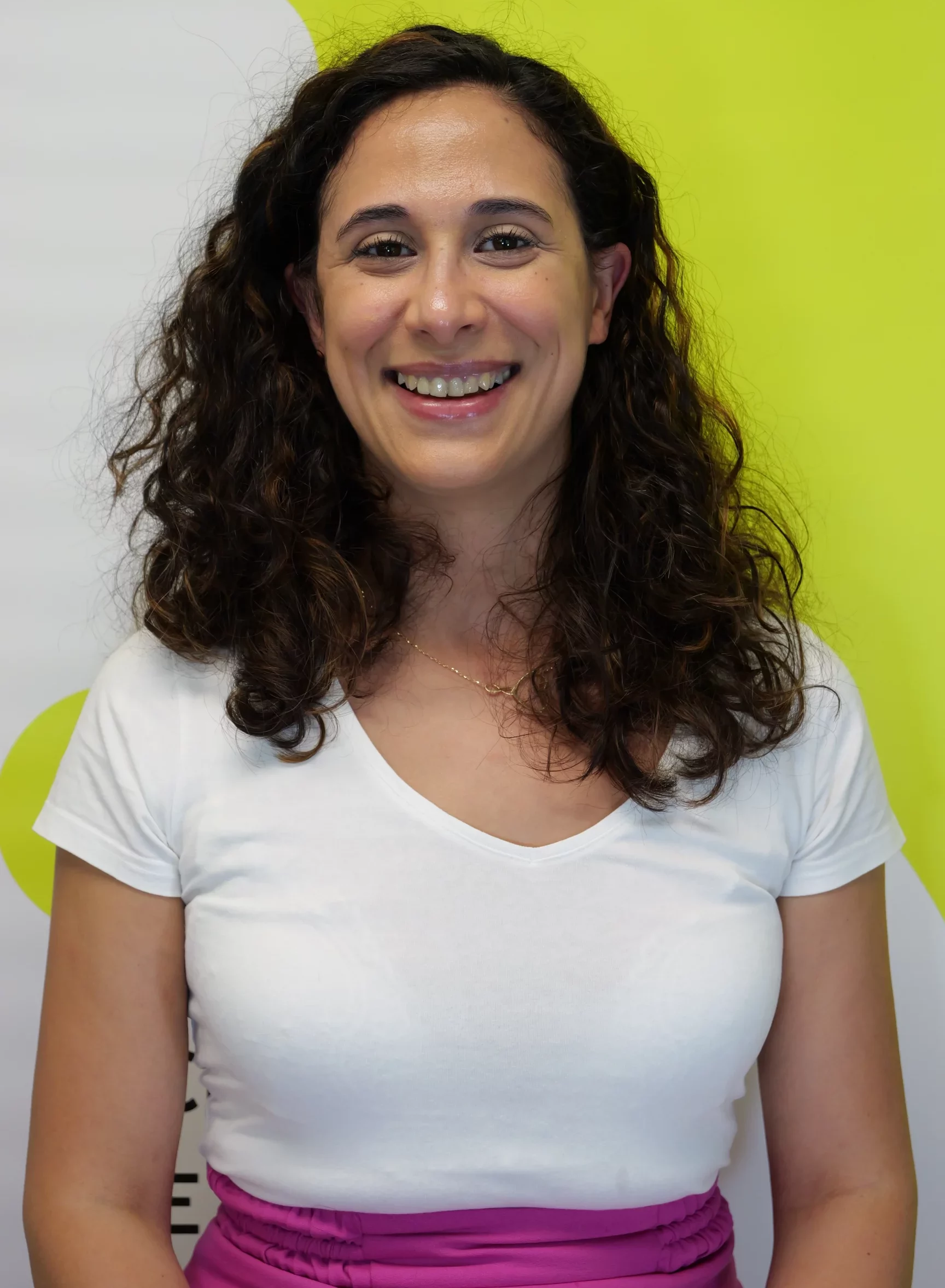
References
- Alavi, S.E., et al., Microfluidics for personalized drug delivery. Drug Discovery Today, 2024. 29(4): p. 103936.
- Galan, E.A., et al., Intelligent Microfluidics: The Convergence of Machine Learning and Microfluidics in Materials Science and Biomedicine. Matter, 2020. 3(6): p. 1893-1922.
- Park, J., Y.W. Kim, and H.-J. Jeon, Machine Learning-Driven Innovations in Microfluidics. Biosensors, 2024. 14(12): p. 613.
- McIntyre, D., et al., Machine learning for microfluidic design and control. Lab on a Chip, 2022. 22(16): p. 2925-2937.
- Chen, L., et al., Review of Image Classification Algorithms Based on Convolutional Neural Networks. Remote Sensing, 2021. 13(22): p. 4712.
- Anowar, F., S. Sadaoui, and B. Selim, Conceptual and empirical comparison of dimensionality reduction algorithms (PCA, KPCA, LDA, MDS, SVD, LLE, ISOMAP, LE, ICA, t-SNE). Computer Science Review, 2021. 40: p. 100378.
- Zhou, J., et al., High-throughput microfluidic systems accelerated by artificial intelligence for biomedical applications. Lab on a Chip, 2024. 24(5): p. 1307-1326.
- Haenlein, M. and A. Kaplan, A brief history of artificial intelligence: On the past, present, and future of artificial intelligence. California management review, 2019. 61(4): p. 5-14.
- Jogin, M., et al. Feature extraction using convolution neural networks (CNN) and deep learning. in 2018 3rd IEEE international conference on recent trends in electronics, information & communication technology (RTEICT). 2018. IEEE.
- Alzubaidi, L., et al., Review of deep learning: concepts, CNN architectures, challenges, applications, future directions. Journal of Big Data, 2021. 8(1): p. 53.
- Malla, R., et al., Revitalizing Cancer Treatment: Exploring the Role of Drug Repurposing. Cancers, 2024. 16: p. 1463.
- Sun, H., et al., Deep learning with microfluidics for on-chip droplet generation, control, and analysis. Frontiers in Bioengineering and Biotechnology, 2023. 11: p. 1208648.
- De Almeida, A.F., R. Moreira, and T. Rodrigues, Synthetic organic chemistry driven by artificial intelligence. Nature Reviews Chemistry, 2019. 3(10): p. 589-604.
- Sun, H., et al., Integrated smart analytics of nucleic acid amplification tests via paper microfluidics and deep learning in cloud computing. Biomedical Signal Processing and Control, 2023. 83: p. 104721.
- Abe, T., S. Oh-Hara, and Y. Ukita, Integration of reinforcement learning to realize functional variability of microfluidic systems. Biomicrofluidics, 2022. 16(2).
- Shayan, M., et al., Toward secure microfluidic fully programmable valve array biochips. IEEE Transactions on Very Large Scale Integration (VLSI) Systems, 2019. 27(12): p. 2755-2766.
- Mahdi, Y. and K. Daoud, Microdroplet size prediction in microfluidic systems via artificial neural network modeling for water-in-oil emulsion formulation. Journal of Dispersion Science and Technology, 2017. 38(10): p. 1501-1508.
- Lashkaripour, A., et al., Design automation of microfluidic single and double emulsion droplets with machine learning. Nature Communications, 2024. 15(1): p. 83.
- Lashkaripour, A., et al., Machine learning enables design automation of microfluidic flow-focusing droplet generation. Nature communications, 2021. 12(1): p. 25.
- Zhang, N., Z. Liu, and J. Wang, Machine-learning-enabled design and manipulation of a microfluidic concentration gradient generator. Micromachines, 2022. 13(11): p. 1810.
- Hadikhani, P., et al., Learning from droplet flows in microfluidic channels using deep neural networks. Scientific reports, 2019. 9(1): p. 8114.
- Pouyanfar, N., et al., Artificial intelligence-based microfluidic platforms for the sensitive detection of environmental pollutants: Recent advances and prospects. Trends in Environmental Analytical Chemistry, 2022. 34: p. e00160.
- Ao, Z., et al., Microfluidics guided by deep learning for cancer immunotherapy screening. Proceedings of the National Academy of Sciences, 2022. 119(46): p. e2214569119.
- Munoz, H.E., et al., Fractal LAMP: Label-free analysis of fractal precipitate for digital loop-mediated isothermal nucleic acid amplification. ACS sensors, 2020. 5(2): p. 385-394.
- Alvarez, M., et al., Enhancing droplet-based single-nucleus RNA-seq resolution using the semi-supervised machine learning classifier DIEM. Scientific reports, 2020. 10(1): p. 11019.
- Song, Y., et al., Machine learning-based cytokine microarray digital immunoassay analysis. Biosensors and Bioelectronics, 2021. 180: p. 113088.
- Lin, S., et al., An ultralight, flexible, and biocompatible all-fiber motion sensor for artificial intelligence wearable electronics. npj Flexible Electronics, 2022. 6(1): p. 27.
- Zhang, Y. and T.H. Tao, Skin‐friendly electronics for acquiring human physiological signatures. Advanced Materials, 2019. 31(49): p. 1905767.
- Zhou, Y., et al., Intelligent classification of platelet aggregates by agonist type. Elife, 2020. 9: p. e52938.
- Rauf, S., et al., Digital E. coli counter: a microfluidics and computer vision-based DNAzyme method for the isolation and specific detection of E. coli from water samples. Biosensors, 2022. 12(1): p. 34.
- Liu, Y., S. Li, and Y. Liu, Machine Learning-Driven Multiobjective Optimization: An Opportunity of Microfluidic Platforms Applied in Cancer Research. Cells, 2022. 11(5).
- Wu, Y., et al., Intelligent frequency-shifted optofluidic time-stretch quantitative phase imaging. Optics Express, 2020. 28(1): p. 519-532.
- Uslu, F., et al., Automated quantification of immunomagnetic beads and leukemia cells from optical microscope images. Biomedical Signal Processing and Control, 2019. 49: p. 473-482.
- Rossi, D., et al., CD4+ versus CD8+ T-lymphocyte identification in an integrated microfluidic chip using light scattering and machine learning. Lab on a Chip, 2019. 19(22): p. 3888-3898.
- Feng, Y., et al., Neural network-enhanced real-time impedance flow cytometry for single-cell intrinsic characterization. Lab on a Chip, 2022. 22(2): p. 240-249.
- Heo, Y.J., et al., Real-time image processing for microscopy-based label-free imaging flow cytometry in a microfluidic chip. Scientific reports, 2017. 7(1): p. 11651.
- Shahab, M. and R. Rengaswamy, Reinforcement-Learning designs droplet microfluidic networks. Computers & Chemical Engineering, 2022. 161: p. 107787.
- Tsai, H.F., S. Podder, and P.Y. Chen, Microsystem Advances through Integration with Artificial Intelligence. Micromachines (Basel), 2023. 14(4).